Articles
Name | Author | |
---|---|---|
How I See IT: What’s in your solution blueprint | Allan Bachan VP, Managing Director, MRO Operations, ICF. | View article |
Teaching machines to better identify chronic defects | Mark Langley, Chief Technology Officer, ATP CaseBank | View article |
Artificial Intelligence Applications and Advanced Analytics in Aviation Operation | Jahan Alamzad, President, Laminaar Aviation InfoTech Americas, Inc. | View article |
Case Study: Air France – KLM Group – Digitization of Cabin Maintenance | Remco Groeneweg, Project Manager, Air France - KLM Group IT; Michael Lopez, Sales Manager, AirInt Services | View article |
Teaching machines to better identify chronic defects
Author: Mark Langley, Chief Technology Officer, ATP CaseBank
Subscribe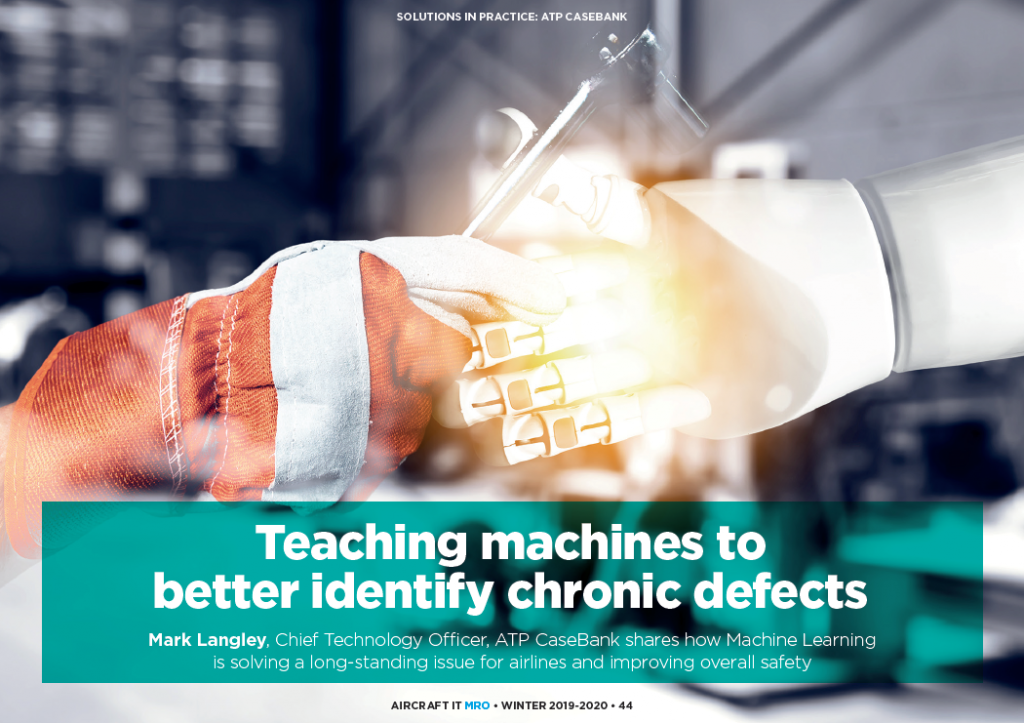
Mark Langley, Chief Technology Officer, ATP CaseBank shares how Machine Learning is solving a long-standing issue for airlines and improving overall safety
Even the most modern of commercial aircraft are not immune to technical faults. Every plane will have something to report error wise during its gate-to-gate voyage, and airlines are accustomed to plowing through all of the data they receive across their entire fleet to find problems. This data is critical for airlines to understand and diagnose potential safety issues before something more serious occurs. In this article, we look at the impact these error codes have on safety, and how advancements in natural language processing and machine learning is helping technicians to improve the accuracy with which airlines are reviewing and assessing their impact.
A LONG-STANDING AIRLINE INDUSTRY PROBLEM
A medium-sized airline fleet can produce nearly 1,000 new records each day, with roughly 40% of the defects not being flagged correctly to maintenance control. The reason for this is that most defects are being reported under incorrect Air Transport Association (ATA) codes, along with general flaws in the system.
Technical faults need to be reported and pilots, aircraft maintenance technicians, and engineers are required to use the ATA codes properly to identify problems. Line mechanics and engineers also refer to the ATA codes in looking up technical data for troubleshooting any given fault. This is a manual process that leads to errors. Given the large number of ATA code combinations and permutations, it is not possible for mechanics to remember all of them. As a result, often the codes for lesser known faults are recorded incorrectly. Since technicians are pressed to dispatch and repair the aircraft in a timely manner, another common issue is the challenge of correctly identifying and entering the proper ATA code simply due to bandwidth. For a variety of reasons, repeat defects often go unnoticed.
The correct identification of repeat defects is very important as technicians, when dealing with them, are required to be more thorough and spend more time to accurately troubleshoot and repair the fault. When an ATA code is entered incorrectly, it not only prevents the detection of a repeat defect, but it may also trigger a false positive, as the incorrectly coded defect may be grouped with an entirely unrelated defect.
Another critical issue with this process is the amount of time it takes to identify and manage repeat defects. The details of the fault have to be transcribed manually from the paper log into the airline’s maintenance and engineering IT (M&E) system. Time-wise, this could take anywhere from one hour to two days. This is intensified by the time it takes technicians in maintenance control to identify ATA defects that are incorrectly logged, as often the same fault occurs many times but a different ATA code is reported for each. Correction of these errors can take up to 30 days to resolve, if they are identified.
HOW TECHNOLOGY CAN HELP
The team responsible for the ChronicX® Recurring Defect Management solution, in use by 25% of the world’s commercial aircraft fleet, identified this as being an issue with their clients early on. Their software helps improve the health and safety of fleets by quickly and accurately identifying and managing recurring or chronic defects across all aircraft, regardless of the assigned ATA coding. ChronicX® bridges the gap between the inconsistent reporting of faults and defects in the aircraft’s technical log by mechanics, and the information that gets entered into the M&E system, giving airlines the ability to dramatically improve aircraft reliability.
The solution performs textual analysis of maintenance logbook reports, to identify similar descriptions written by different line mechanics. It can be used to help match two faults that were entered using different codes. Using natural language processing (NLP), it can analyze and categorize large amounts of text data and understand similarities. Recently, the development team for ChronikX® revealed a new feature that has the ability to determine and assign the correct ATA code to a defect record.
USING MACHINE LEARNING TO IMPROVE ACCURACY AND OVERALL SAFETY
Maintenance logbooks contain very short text that is full of a technician’s abbreviations and misspellings – not uncommon for them, given the short amount of time they have between aircraft. By using machine learning, the ChronicX® solution noted above has the capability to expand its own library of aviation related acronyms, abbreviations, and synonyms. Recording synonyms is important because working on raw text alone could result in a lot of words being missed. Synonym replacement means that equipment with many colloquial names can still be identified and matched. The more the system is used, the better it’s capability for identifying and matching defect records, even if their ATA coding is incorrect.
The ChronicX® ATA recoding feature automatically predicts the right 4-digit ATA code for a defect, based on its defect description. In addition to providing 4-digit accuracy at a 90%+ level, it continuously learns from the user feedback and thereby its prediction accuracy increases exponentially with continued usage. Such a powerful machine-learning based approach allows users to recode all their defects with reduced effort, and in a fraction of the time it would take with other systems and processes. The time it saves maintenance control analysts means that specialist personnel can focus on more critical and costly fleet maintenance issues.
The industry has been suffering with the problem of incorrect and inconsistent ATA codes being assigned to defects for a long time. With correct ATA codes being assigned at this new degree of accuracy, airlines can increase the accuracy of their overall data required for analysis, planning, and improvement of aircraft maintenance in general. Capturing repeat defects earlier leads to better on-time departure times, reduced delays, and fewer flight cancellations. Further, when combined with a recurring defect analysis solution, it contributes towards overall fleet safety by accurately helping to understand the health of the entire fleet, and individual aircraft, in real-time.
Contributor’s Details
Mark Langley
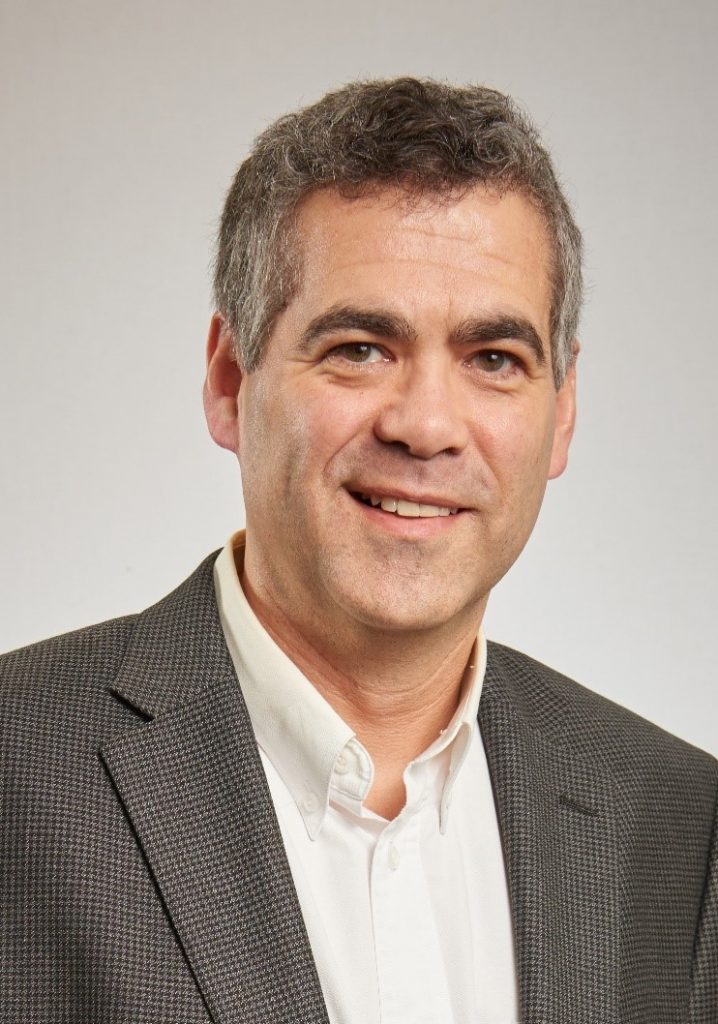
Mark was a co-founder of CaseBank and architect of its SpotLight product family. He led software development, product design, and technical sales support as the company grew, culminating as VP R&D. Currently, as CTO, Mark sets the technical vision, roadmap, and requirements for ATP’s product offerings. He enjoys discovering and evaluating technical solutions to business needs and fostering technical excellence throughout the company’s operations. Mark studied Engineering Science (Aerospace option) at University of Toronto.
ATP CaseBank
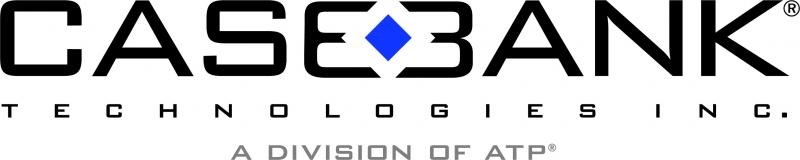
CaseBank Technologies Inc., a Division of ATP, develops innovative software solutions for troubleshooting and identifying recurring defects on aircraft and other complex equipment. Using case-based reasoning, natural language processing and machine learning, CaseBank’s systems accelerate and improve the accuracy of operations, service and support decisions.
Comments (0)
There are currently no comments about this article.
To post a comment, please login or subscribe.